Dynamics of Texas’ Rural Land Market
A model of Texas’ rural land markets reveals dynamics similar to those observed in general investment markets. These results contrast with the conventional paradigm focused on the illiquid and inflation-hedging qualities of rural land markets.
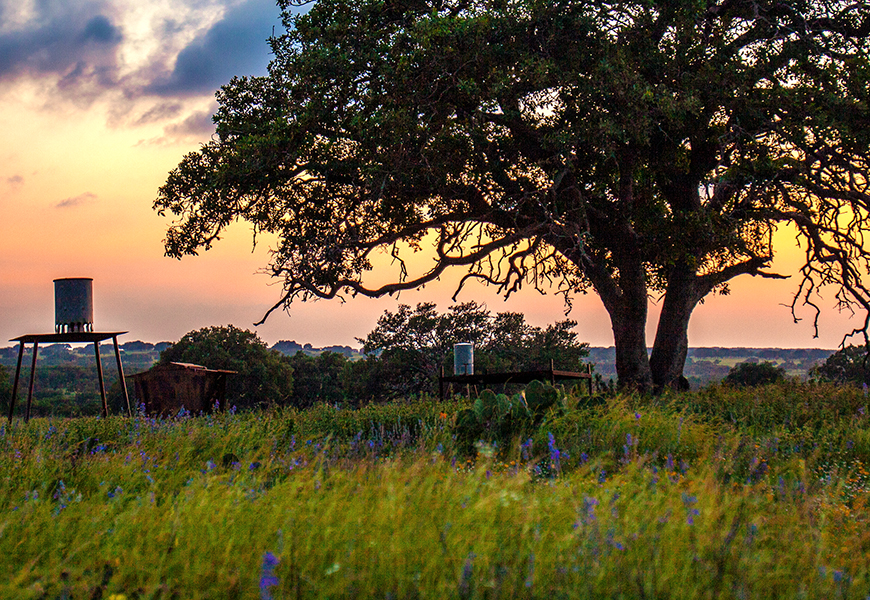
Dynamics of Texas’ Rural Land Market
A model of Texas’ rural land markets reveals dynamics similar to those observed in general investment markets. These results contrast with the conventional paradigm focused on the illiquid and inflation-hedging qualities of rural land markets. The model demonstrates general macroeconomic factors drive Texas’ rural land market trends, not the usual rural or agricultural financial drivers examined in most research.
This aggregate, multivariate econometric model describes land prices and sales volume not as a function of net farm income but as a function of Texas’ total personal income, the fed funds rate, and oil prices. Of those market influences, oil prices contribute the most uncertainty. Knowledge that rural land markets are decoupled from traditional agricultural influences should inspire state and local policymakers to rethink conventional assumptions about the effectiveness of measures targeting rural land markets and landowners.
This research strives to (1) explain the aggregate dynamics of Texas land markets, (2) identify the most important economic indicators of land market dynamics, (3) analyze the impacts of U.S. macroeconomic and monetary policies on these market drivers, and (4) analyze the land market business cycle.
Section 1 reviews land market modeling literature. Section 2 introduces the Texas rural land market model. Section 3 presents empirical results. Section 4 presents the results of simulations using the estimated model to forecast impacts of changes in U.S. monetary policy rates on the state’s rural land market. Section 5 concludes with a summary of the findings.
Land Market Modeling Literature
Many analysts have provided insights into land markets focusing primarily on prices related to either microeconomic or macroeconomic exogenous drivers of markets. For example, Alston (1986) focused on macroeconomic factors to try to explain dramatic changes in cropland prices. Specifically, he focused on the relationship between inflation and cropland prices. Buyers, he hypothesized, considered hedging opportunities against inflation by investing in cropland. Using a tightly parameterized empirical model, he concluded there is no support for the notion of cropland as a hedge for inflation and that net rental income was the primary driver for most of the real growth in U.S. land prices during the 20 years prior to 1982.
Richard E. Just and John A. Miranowski attempted to explain changes in cropland prices using previous literature’s determinants. The authors identify the relative roles of each models’ influence using mainly a theoretical framework with some empirical analysis. They consider farm and nonfarm returns, inflation, credit, the real interest rate on farm real estate debt, government payments, taxation, and more. Their results indicate inflation, net returns, and the discount rate are the largest contributors. Using these results, Just and Miranowski found they could successfully predict land prices using current and lagged rental rates, with lags of up to eight years. They note that while lagging rent by those amounts proves effective, it lacks substance in terms of “defensible economic rationale.” Allen M. Featherstone and Timothy G. Baker and, in a separate study, Barry Falk agree that these techniques, while proving strong in terms of an r-squared, are not applicable.
Gregory Ibendahl and Terry Griffin provide intuition on why the lags are important in determining the land price. They explain how an asymmetric relationship exists between changes in rent and land prices. The asymmetries are created when the lessee chooses to share varying amounts of information during productive and non-productive years. The authors determine this asymmetric relationship between lessee and landowner is the basis for the need to use lags in determining land prices.
Emanuel Melichar originated the use of cash rent as a proxy for income, placing emphasis on expected changes in rental rates. His work arose from the rapid increases in farm asset prices. He noted the importance of understanding where the capital gains are occurring—from the return to the asset or other influences. He states the increases in returns to land over labor are the primary cause of the increases in land prices. “Over the last 25 years, the proportion of the total return that could be ascribed to operators’ labor has dropped from 63 percent to 17 percent, while the proportion that could be regarded as a return to production assets has risen from 25 percent to 69 percent,” he wrote.
To properly assess the income to land price relationship, Melichar states net farm income cannot be reliably used for several reasons. First, net farm income is an aggregate measure, whereas land prices are a unit price. Using net farm income to represent return to land alone ignores the number of other productive assets that are included in the net farm income measure. Additionally, net farm income lacks comprehension of the non-operator landowner. When considering the substantial portion of land owned by non-operators, income from rent and interest payments on debt should be included in income while operator’s dwellings should be excluded.
Lindon J. Robison, David A. Lins, and Ravi Venkataraman try to improve on the capitalized valuation model by including the expectations of changes in factors. The growth rate in cash returns to land, inflation expectations, income, and capital gains taxes are included in their model. They determine that inflation and non-agricultural demand are important influencers. Similarly, Charles B. Moss considers the effects of returns, interest rates, and inflation on cropland values, concluding inflation is the biggest driver of land values.
Andrew Schmitz takes a closer look at the involvement of government policy and its effect on value with a focus specifically on boom-and-bust cycles. He finds that cropland values and inflation are positively related. He states these results show inflation was a significant contributor to the rise in cropland prices in the 1970s and 1980s.
However, these analyses approach the land value question with farmers as the sole end users of the land. This approach may adequately characterize cropland in remote locations; however, as Ian W. Hardie, Tulika A. Narayan, and Bruce L. Gardner noted, other sources of demand can impact land prices. Specifically, land prices in the mid-Atlantic area were more closely linked to house prices in Baltimore than net farm income. Allison Borchers, Jennifer Ifft, and Todd Kuethe explored the role of use values and amenities in setting prices, concluding farmland values are “only partially explained by agricultural returns.” Other current and potential future uses substantially impacted land price trends, meaning non-agricultural influences are likely important influences on values.
Research published by Wendong Zhang and C.J. Nickerson studies land values in relation to distance to urban centers and housing market prices. They found that the 2008-09 housing bust negatively impacted farmland prices as demand from urban-based potential users of land temporarily evaporated. These results suggest the market for land presides over a contest between agriculturally and non-agriculturally based buyers.
These latest studies recognize the complex nature of land markets where multiple potential uses compete for space. The market ebbs and flows with the fortunes of potential users. This means levels of personal income influence demand from all sectors of the economy by expanding available funds for land purchases. Oil prices play an important role in Texas by providing extra income to current landowners. This study focuses on the land market as a complex of interrelated relationships driving the demand for and supply of land as they interact to set prices paid and quantities exchanged in Texas rural land markets.
Texas Rural Land Market Model
The Texas rural land database includes all reported sales not involved in urban-style developments, greater than regional minimum acreages ranging from 45 to 160 acres, and with prices less than $30,000 per acre. These criteria, along with location and current use, identify the sales as rural in character, so they exclude transitional or development tracts. Frequently observed uses consist of recreation, farming, ranching, natural resource extraction, and timber production. This market data constitute a quarterly time series of both quantities and prices of rural land sold starting in first quarter 1966. The Texas land market dataset reflects an analysis of information reported by a network of corresponding market observers to the Texas Real Estate Research Center (TRERC) at Texas A&M University.
The reports provide quarterly annualized price and acreage statistics for individual transactions across Texas. The published data present prices based on the studied transactions. Market statistics report prices adjusted for variations in size distribution for each of seven regional markets across the periods studied. The resulting time series for Texas consists of a weighted average of regional prices and total acres traded.
The reported transactions include a mixture of land uses—farming, ranching, wildlife management, minerals, etc. Therefore, statistics in the datasets reflect overall market conditions without regard to a specific land use. For a detailed discussion of the data, see “Using the Center’s Rural Land Market Data.”
Applying Granger causality tests to land price and land sale volume variables and a selection of economic indicators showed that, in addition to their own dynamics, total personal income in Texas, crude oil prices, and fed funds rate drive aggregate land market prices and quantities traded. Using these time series data, TRERC estimated a multivariate econometric model of the state’s rural land market.
The model consists of five equations representing the demand for and supply of land. The endogenous variables (prices and quantities of land sold) depend on the exogenously determined fed fund rates, Texas aggregate incomes, and oil prices. After testing several models using these variables both adjusted and unadjusted for inflation, TRERC found nominal values generate the most plausible results. This suggests agents in this market make buying and selling decisions based on nominal dollars, rather than real, inflation-adjusted dollars. That means money illusion exists in this market.1 Study results also show land price stickiness plus long memory impulse response functions for land prices, supporting the suspected presence of money illusion, according to E. Shafir, P. Diamond, and A. Tversky. Consequently, the selected final model relied on nominal values of land prices, incomes, and oil prices.
To see this entire report with formulas, figures, and tables, click the download button below.
____________________
1 Money illusion according to Fisher (1928) is widespread as many people think in nominal rather than real terms. See also Shafir, Diamond, and Tversky, A. (1997).
You might also like

Publications
Receive our economic and housing reports and newsletters for free.